Interpretable bias mitigation for textual data: Reducing gender bias in patient notes while maintaining classification performance
J. R. Minot, N. Cheney, M. Maier, D. Elbers, C. M. Danforth, and P. S. Dodds
ACM Transactions on Computing for Healthcare, 3, 1–41, 2022
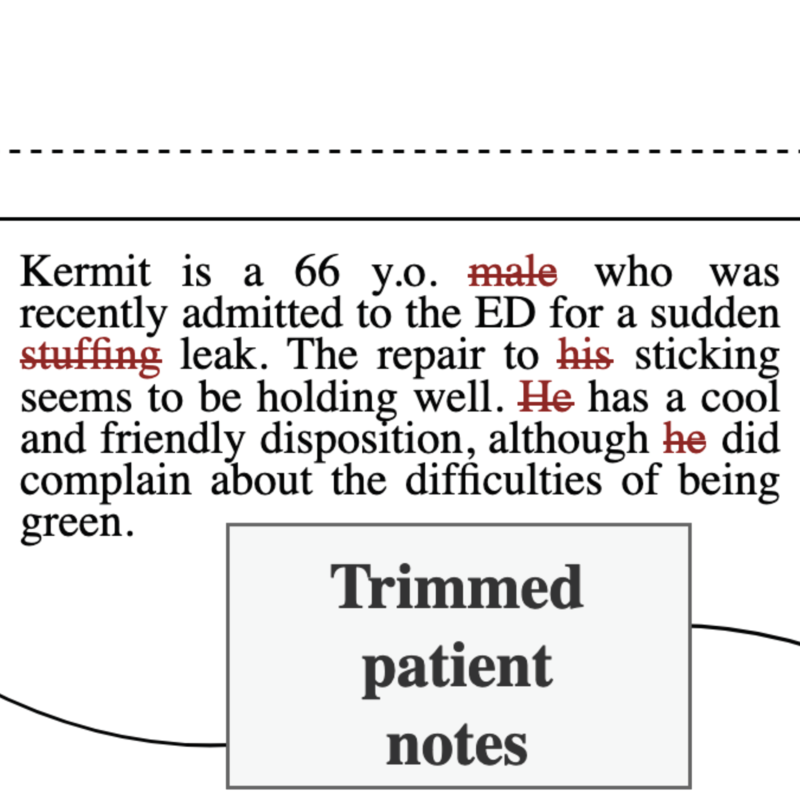
Times cited: 22
Abstract:
Medical systems in general, and patient treatment decisions and outcomes in particular, are affected by bias based on gender and other demographic elements. As language models are increasingly applied to medicine, there is a growing interest in building algorithmic fairness into processes impacting patient care. Much of the work addressing this question has focused on biases encoded in language models—statistical estimates of the relationships between concepts derived from distant reading of corpora. Building on this work, we investigate how word choices made by healthcare practitioners and language models interact with regards to bias. We identify and remove gendered language from two clinical-note datasets and describe a new debiasing procedure using BERT-based gender classifiers. We show minimal degradation in health condition classification tasks for low- to medium-levels of bias removal via data augmentation. Finally, we compare the bias semantically encoded in the language models with the bias empirically observed in health records. This work outlines an interpretable approach for using data augmentation to identify and reduce the potential for bias in natural language processing pipelines.
- This is the default HTML.
- You can replace it with your own.
- Include your own code without the HTML, Head, or Body tags.
BibTeX:
@Misc{minot2022a, author = {Minot, Joshua R. and Cheney, Nick and Maier, Marc and Elbers, Danne and Danforth, Christopher M. and Dodds, Peter Sheridan}, title = {Interpretable bias mitigation for textual data: {R}educing gender bias in patient notes while maintaining classification performance}, journal = {ACM Transactions on Computing for Healthcare}, year = {2022}, volume = {3}, pages = {1–41}, note = {Available online at \href{https://arxiv.org/abs/2103.05841}{https://arxiv.org/abs/2103.05841}}, }