Sentiment analysis of medical record notes for lung cancer patients at the Department of Veterans Affairs
D. Elbers, J. La, J. R Minot, R. E. Gramling, M. T. Brophy, N. V. Do, N. Fillmore, P. S. Dodds, C. M. Danforth
PLOS ONE, 18, e0280931, 2023
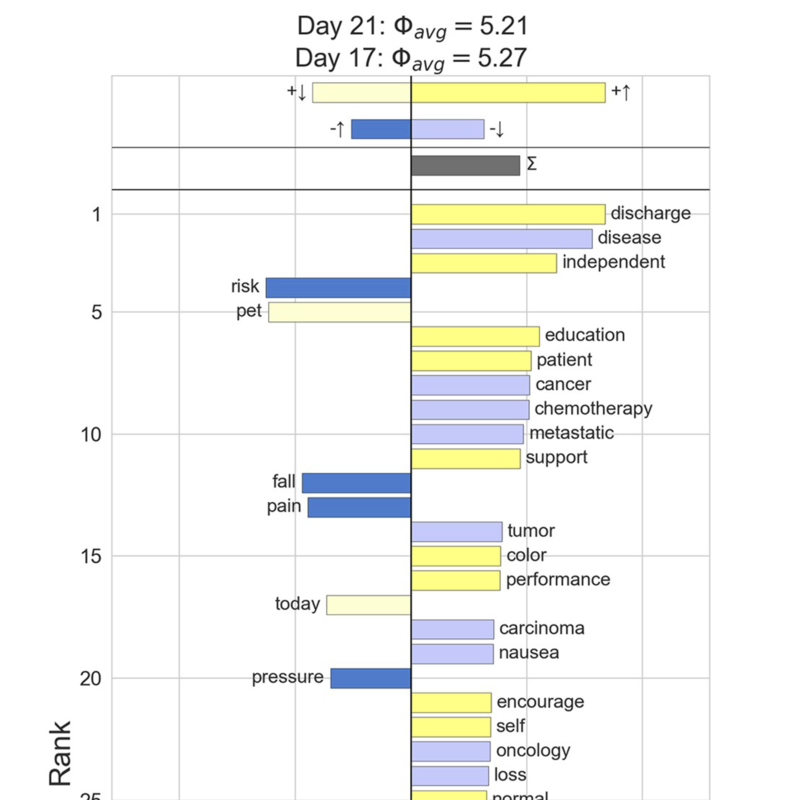
Times cited: 0
Abstract:
Natural language processing of medical records offers tremendous potential to improve the patient experience. Sentiment analysis of clinical notes has been performed with mixed results, often highlighting the issue that dictionary ratings are not domain specific. Here, for the first time, we re-calibrate the labMT sentiment dictionary on 3.5M clinical notes describ- ing 10,000 patients diagnosed with lung cancer at the Department of Veterans Affairs. The sentiment score of notes was calculated for two years after date of diagnosis and evaluated against a lab test (platelet count) and a combination of data points (treatments). We found that the oncology specific labMT dictionary, after re-calibration for the clinical oncology domain, produces a promising signal in notes that can be detected based on a comparative analysis to the aforementioned parameters.
- This is the default HTML.
- You can replace it with your own.
- Include your own code without the HTML, Head, or Body tags.
BibTeX:
@Article{elbers2023a, author = {Elbers, Danne C. and La, Jennifer and Minot, Joshua R. and Gramling, Robert and Brophy, Mary T. and Do, Nhan V. and Fillmore, Nathanael R. and Dodds, Peter Sheridan and Danforth, Christopher M.}, title = {Sentiment analysis of medical record notes for lung cancer patients at the {D}epartment of {V}eterans {A}ffairs}, journal = {PLOS ONE}, year = {2023}, key = {health,people,language,text,happiness}, volume = {18}, pages = {e0280931}, }