Long-term word frequency dynamics derived from Twitter are corrupted: A bespoke approach to detecting and removing pathologies in ensembles of time series
P. S. Dodds, J. R. Minot, M. V. Arnold, T. Alshaabi, J. L. Adams, D. R. Dewhurst, A. J. Reagan, and C. M. Danforth
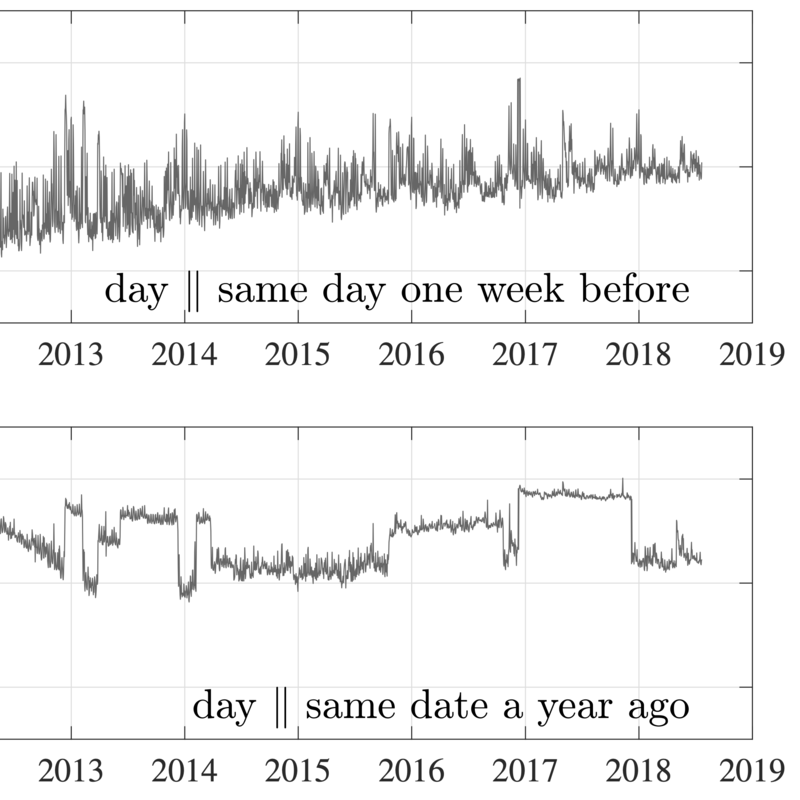
Times cited: 6
Abstract:
Maintaining the integrity of long-term data collection is an essential scientific practice. As a field evolves, so too will that field's measurement instruments and data storage systems, as they are invented, improved upon, and made obsolete. For data streams generated by opaque sociotechnical systems which may have episodic and unknown internal rule changes, detecting and accounting for shifts in historical datasets requires vigilance and creative analysis. Here, we show that around 10\% of day-scale word usage frequency time series for Twitter collected in real time for a set of roughly 10,000 frequently used words for over 10 years come from tweets with, in effect, corrupted language labels. We describe how we uncovered problematic signals while comparing word usage over varying time frames. We locate time points where Twitter switched on or off different kinds of language identification algorithms, and where data formats may have changed. We then show how we create a statistic for identifying and removing words with pathological time series. While our resulting process for removing 'bad' time series from ensembles of time series is particular, the approach leading to its construction may be generalizeable.
- This is the default HTML.
- You can replace it with your own.
- Include your own code without the HTML, Head, or Body tags.
BibTeX:
@Misc{dodds2020c, author={Dodds, Peter Sheridan and others}, title={Long-term word frequency dynamics derived from {T}witter are corrupted: {A} bespoke approach to detecting and removing pathologies in ensembles of time series}, year={2020}, title = {Long-term word frequency dynamics derived from {T}witter are corrupted: {A} bespoke approach to detecting and removing pathologies in ensembles of time series}, year = {2020}, }