Divergent modes of online collective attention to the COVID-19 pandemic are associated with future caseload variance
D. R. Dewhurst, T. Alshaabi, M. Arnold, J. R. Minot, C. M. Danforth, and P. S. Dodds
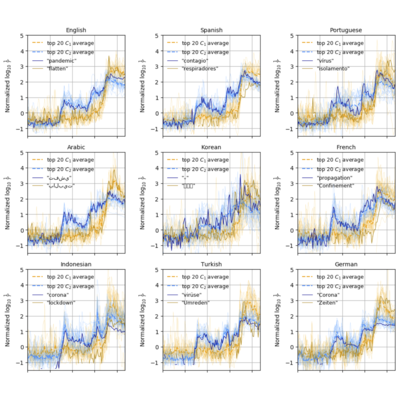
Times cited: 15
Abstract:
Using a random 10\% sample of tweets authored from 2019-09-01 through 2020-03-25, we analyze the dynamic behavior of words (1-grams) used on Twitter to describe the ongoing COVID-19 pandemic. Across 24 languages, we find two distinct dynamic regimes: One characterizing the rise and subsequent collapse in collective attention to the initial Coronavirus outbreak in late January, and a second that represents March COVID-19-related discourse. Aggregating countries by dominant language use, we find that volatility in the first dynamic regime is associated with future volatility in new cases of COVID-19 roughly three weeks (average 22.7 $\pm$ 2.17 days) later. Our results suggest that surveillance of change in usage of epidemiology-related words on social media may be useful in forecasting later change in disease case numbers, but we emphasize that our current findings are not causal or necessarily predictive.
- This is the default HTML.
- You can replace it with your own.
- Include your own code without the HTML, Head, or Body tags.
BibTeX:
@Misc{dewhurst2020a, author = {Dewhurst, David Rushing and Alshaabi, Thayer and Arnold, Michael V. and Minot, Joshua R. and Danforth, Christopher M. and Dodds, Peter Sheridan}, title = {Divergent modes of online collective attention to the {C}{O}{V}{I}{D}-19 pandemic are associated with future caseload variance}, year = {2020}, note = {Available online at \href{https://arxiv.org/abs/2004.03516}{https://arxiv.org/abs/2004.03516}}, }