An evolutionary algorithm approach to link prediction in dynamic social networks
C. A. Bliss, M. R. Frank, C. M. Danforth, and P. S. Dodds
Journal of Computational Science, 5, 750–764, 2014
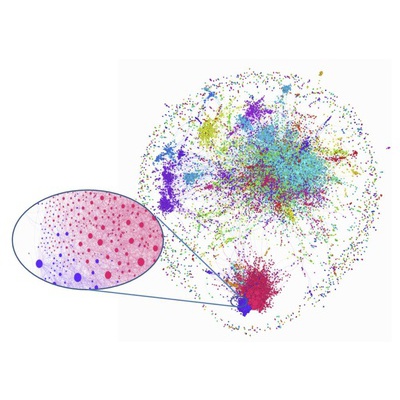
Times cited: 304
Abstract:
Many real world, complex phenomena have underlying structures of evolving networks where nodes and links are added and removed over time. A central scientific challenge is the description and explanation of network dynamics, with a key test being the prediction of short and long term changes. For the problem of short-term link prediction, existing methods attempt to determine neighborhood metrics that correlate with the appearance of a link in the next observation period. Recent work has suggested that the incorporation of user-specific metadata and usage patterns can improve link prediction, however methodologies for doing so in a systematic way are largely unexplored in the literature. Here, we provide an approach to predicting future links by applying an evolutionary algorithm to weights which are used in a linear combination of sixteen neighborhood and node similarity indices. We examine Twitter reciprocal reply networks constructed at the time scale of weeks, both as a test of our general method and as a problem of scientific interest in itself. Our evolved predictors exhibit a thousand-fold improvement over random link prediction with high levels of precision for the top twenty predicted links, to our knowledge strongly outperforming all extant methods. Based on our findings, we suggest possible factors which may be driving the evolution of Twitter reciprocal reply networks.
- This is the default HTML.
- You can replace it with your own.
- Include your own code without the HTML, Head, or Body tags.
Extra: Published online February, 2014.
BibTeX:
@Article{bliss2014b, author = {Bliss, Catherine A. and Frank, Morgan R. and Danforth, Christopher M. and Dodds, Peter Sheridan}, title = {An evolutionary algorithm approach to link prediction in dynamic social networks}, journal = {Journal of Computational Science}, note = {Available online at \href{http://www.sciencedirect.com/science/article/pii/S1877750314000040}{http://www.sciencedirect.com/science/article/pii/S1877750314000040} and at \href{https://arxiv.org/abs/1304.6257}{https://arxiv.org/abs/1304.6257}}, year = {2013}, volume = {}, pages = {}, doi = {DOI: 10.1016/j.jocs.2014.01.003}, key = {networks,prediction}, }