Shadow networks: Discovering hidden nodes with models of information flow
J. P. Bagrow, S. Desu, M. R. Frank, N. Manukyan, L. Mitchell, A. Reagan, E. E. Bloedorn, L. B. Booker, L. K. Branting, M. J. Smith, B. F. Tivnan, C. M. Danforth, P. S. Dodds, and J. C. Bongard
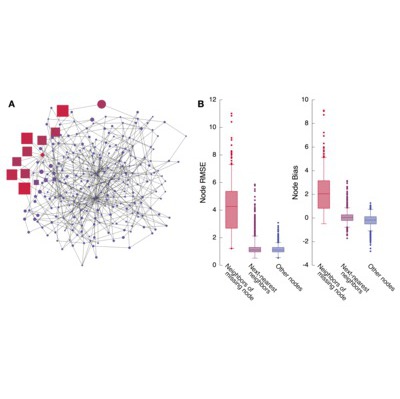
Times cited: 8
Abstract:
Complex, dynamic networks underlie many systems, and understanding these networks is the concern of a great span of important scientific and engineering problems. Quantitative description is crucial for this understanding yet, due to a range of measurement problems, many real network datasets are incomplete. Here we explore how accidentally missing or deliberately hidden nodes may be detected in networks by the effect of their absence on predictions of the speed with which information flows through the network. We use Symbolic Regression (SR) to learn models relating information flow to network topology. These models show localized, systematic, and non-random discrepancies when applied to test networks with intentionally masked nodes, demonstrating the ability to detect the presence of missing nodes and where in the network those nodes are likely to reside.
- This is the default HTML.
- You can replace it with your own.
- Include your own code without the HTML, Head, or Body tags.
BibTeX:
@Misc{bagrow2013a, author = {Bagrow, J.P and Desu, S and Frank, M. R and Manukyan, N and Mitchell, L and Reagan, A and Bloedorn, E.E and Booker, L. B. Branting, L. K and Smith, M. J and Tivnan, B. F and Danforth, C. M and Dodds, P. S and Bongard, J. C.}, title = {Shadow networks: {D}iscovering hidden nodes with models of information flow}, year = {2013}, note = {Preprint available at \href{https://arxiv.org/abs/1312.6122}{https://arxiv.org/abs/1312.6122}}, }