Augmenting semantic lexicons using word embeddings and transfer learning
T. Alshaabi, C. Van Oort, M. I. Fudolig, M. V. Arnold, C. M. Danforth, and P. S. Dodds
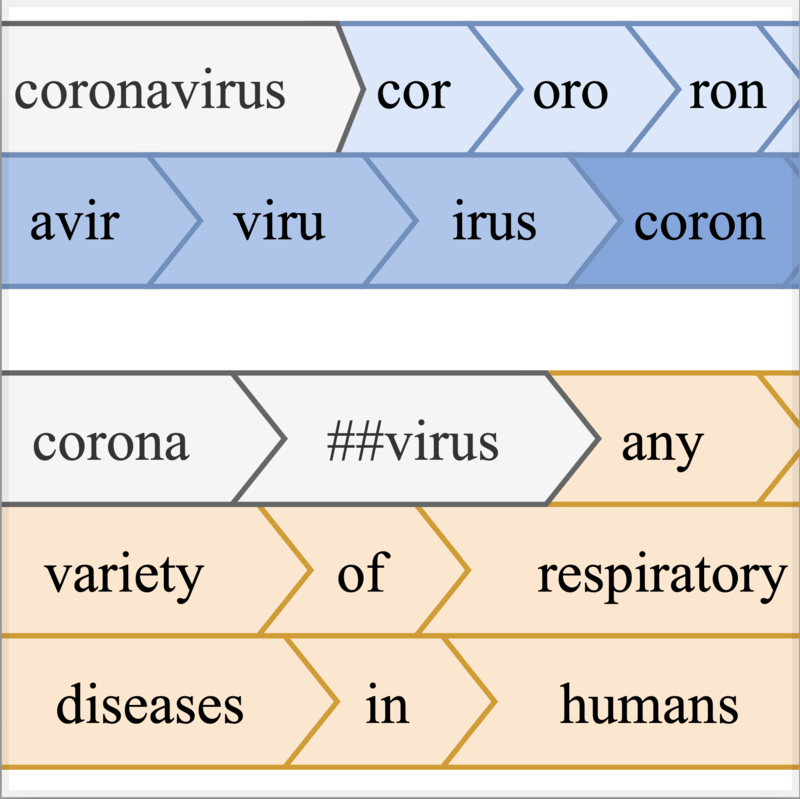
Times cited: 4
Abstract:
Sentiment-aware intelligent systems are essential to a wide array of applications including marketing, political campaigns, recommender systems, behavioral economics, social psychology, and national security. These sentiment-aware intelligent systems are driven by language models which broadly fall into two paradigms: 1. Lexicon-based and 2. Contextual. Although recent contextual models are increasingly dominant, we still see demand for lexicon-based models because of their interpretability and ease of use. For example, lexicon-based models allow researchers to readily determine which words and phrases contribute most to a change in measured sentiment. A challenge for any lexicon-based approach is that the lexicon needs to be routinely expanded with new words and expressions. Crowdsourcing annotations for semantic dictionaries may be an expensive and time-consuming task. Here, we propose two models for predicting sentiment scores to augment semantic lexicons at a relatively low cost using word embeddings and transfer learning. Our first model establishes a baseline employing a simple and shallow neural network initialized with pre-trained word embeddings using a non-contextual approach. Our second model improves upon our baseline, featuring a deep Transformer-based network that brings to bear word definitions to estimate their lexical polarity. Our evaluation shows that both models are able to score new words with a similar accuracy to reviewers from Amazon Mechanical Turk, but at a fraction of the cost.
- This is the default HTML.
- You can replace it with your own.
- Include your own code without the HTML, Head, or Body tags.
BibTeX:
@Misc{alshaabi2021e, author = {Alshaabi, Thayer and Van Oort, Colin and Fudolig, Mikaela Irene and Arnold, Michael V. and Danforth, Christopher M. and Dodds, Peter Sheridan}, title = {Augmenting semantic lexicons using word embeddings and transfer learning}, year = {2021}, key = {language}, note = {Available online at \href{https://arxiv.org/abs/2109.09010}{https://arxiv.org/abs/2109.09010}}, }